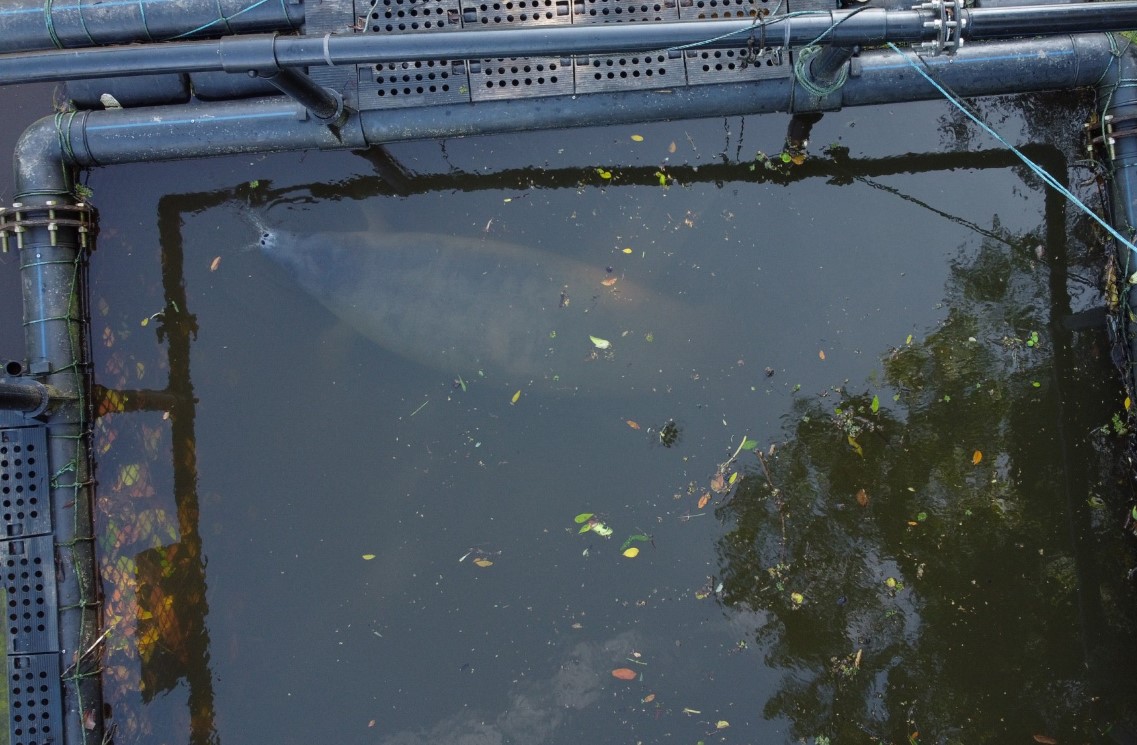
Fernando Merchan, Fernando Merchan, Kenji Contreras, Hector Poveda, Hector M. Guzman, Javier E. Sanchez-Galan
Published: August 08, 2024
Introduction: This work presents a methodology based on unsupervised learning to identify and count unique manatees using underwater vocalization recordings.
Methods: The proposed approach utilizes the Scattering Wavelet Transform (SWT) to represent individual manatee vocalizations. A dimensionality reduction technique, known as PaCMAP, is employed. A density-based algorithm, Hierarchical Density-Based Spatial Clustering of Applications with Noise (HDBSCAN), is used to count and identify groups of individual manatee vocalizations. The proposed methodology is compared with a previous method developed by our group, which used classical clustering methods (K-Means and hierarchical clustering) and spectrograms based on the Short-Time Fourier Transform (STFT) to represent the vocalizations. The performance of both approaches is contrasted using a new vocalization dataset consisting of 23 manatees from the Greater Caribbean, temporarily captured in the San San River, Bocas del Toro, western Panama, as input.
Results: The proposed methodology achieves an average error rate of 14.05% in estimating the number of individuals (i.e., groups) and an 83.75% success rate in correctly grouping a manatee into a cluster.
Discussion: Thus, the proposed approach outperforms our previous analysis methodology for the same dataset. The value of this work lies in providing a way to estimate the manatee population based solely on underwater bioacoustics.
Journal: Frontiers in Marine Science